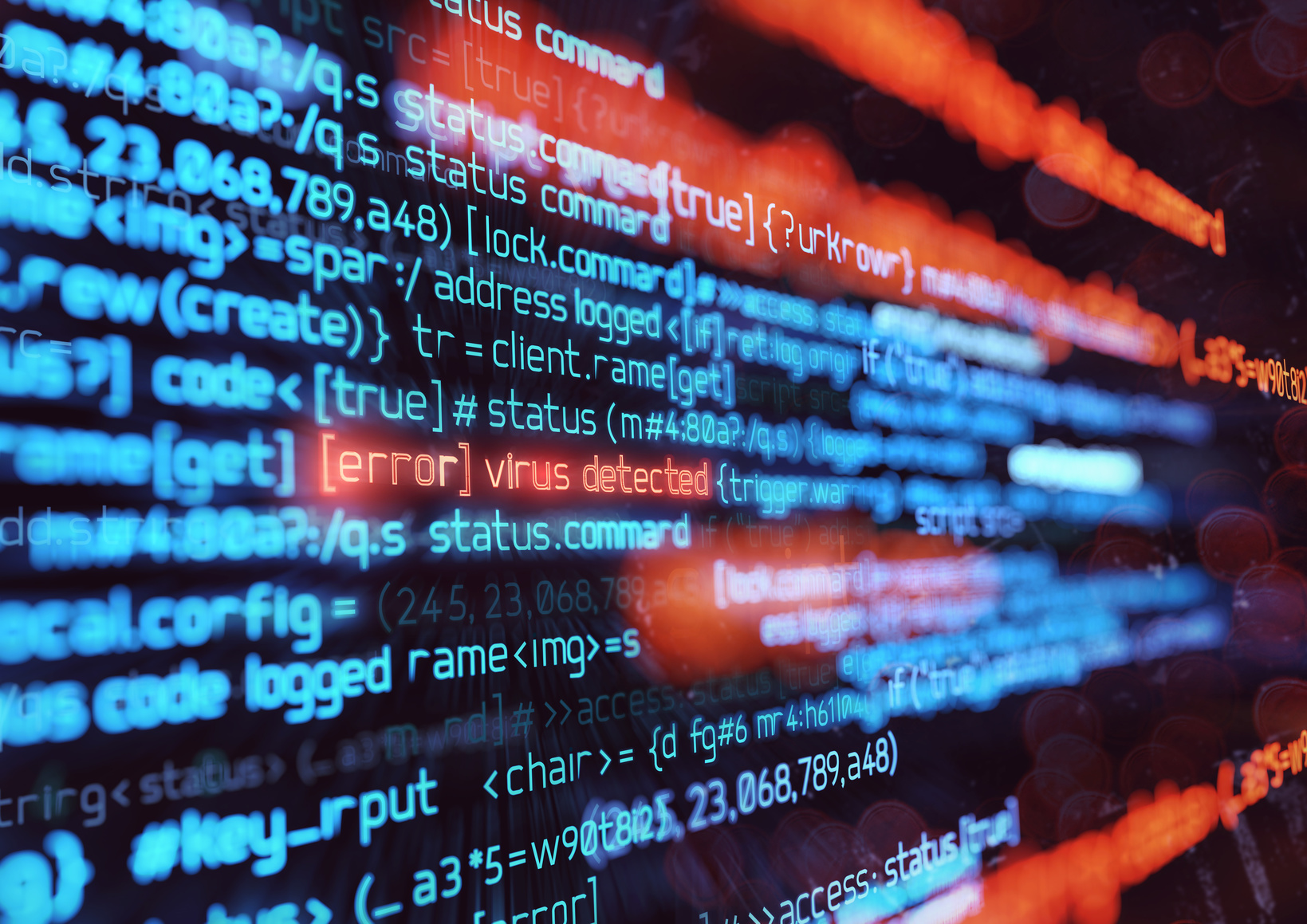
Open Data – challenges and legal strings
Author
Beatrice Huber
NRP 75 “Big Data”
Data sharing is at the heart of future research. Therefore, initiatives promote open data. But reality often looks different as data is often inaccessible, underutilized or in other manners closed off for publicly funded research projects. How can this be made better? This is being taken care of by a cross-cutting activity within NRP 75 that started with a workshop on 18 February in Basel.
Prof. Sabine Gless from the University of Basel whose team initiated the cross-cutting activity explained the motives of the project in her introduction. She is often confronted with the question “Am I allowed to use the data?” and the answer is not that easy. The project wants to map the ground to start a discussion about the legal strings for publicly funded projects.
Christine Möhrke-Sobolewski, one of the researchers working in the project, outlined the challenges. Data is not data and open data is different in every field. Open data has a lot of benefits as with open data knowledge is made more available for everyone. But what about the challenges? There are technical ones like the questions regarding formats and repositories. And time is also a big challenge as well as questions about provenance, social and cultural aspects. And the financial costs. The legal situation also presents some challenges: ownership? licenses? privacy? intellectual property? The workshop is one of the first steps to map the ground for legal strings.
SNSF Open Data Research Policy
Lionel Perini from the SNSF reported on their Open Data Research Policy and the first experiences with data management plans (DMP). The SNSF has a new policy about open data but data sharing requirements are not really new. They value research data sharing as a fundamental contribution to the impact, transparency and reproducibility of scientific research. In addition to being carefully curated and stored, the SNSF believes research data should be as openly as possible.
Therefore, the SNSF expects all its funded researchers to store the research data they have worked on and produced during the course of their research work, to share these data with other researchers, unless they are bound by legal, ethical, copyright, confidentiality or other causes, and to deposit their data and metadata onto existing repositories in formats that anyone can find, access and reuse without restriction. Today a DMP is a formal requirement for every project proposal. Lionel Perini presented the FAIR data principles which asked data to be findable, accessible, interoperable, and reusable. SNSF is promoting a “light version” of the principles. The SNSF published the first monitoring report on DMP (2017‒2018) and the results are encouraging after these two years. Most DMP are accepted, no revisions were necessary in most cases.
Who has which right to research data?
In the next steps of the project guideline-based explorative interviews with experts are planned. Susanne Knickmeier, another researcher from the project team, presented the draft of the questionnaire and the procedure. Experts will be selected based on their research area, but also on their area activity and experience with open data. One of the goals of the workshop was to get input for the questionnaire. What are really the problems, the practical problems? The discussion showed that there is a big difference between quantitative and qualitative data. What exactly is data? Are notes already data?
More input came from the participants. Machine learning has to deal with copyright issues due to the multimedia content they use. In YouTube, for instance, you can give a CC license to your video but YouTube writes in their terms that downloading is not allowed. And multimedia data is very soon personal. Who is responsible or liable for what? Responsibility tends to be passed on. Often researchers hear: “You are not allowed to do it but we will not prosecute you …” In summary machine learning has copyright problems, social sciences have privacy problems. Anonymization is not always a solution as it can take away too much information to make data useless. Standards are a good thing. But there are too many.
Why data property is a very bad idea
At the end of the workshop and as a special input Kirsten Schmidt presented her dissertation on the allocation of personal data. Everyone thinks that Big Data has the big potential for making big money. And for that personal data is the key! Therefore, it is not surprising that some think that “a lot of people are making a lot of money with MY data!” But is that really true? Data has some specialities: There is no competition in use and it is not getting worse by using it. Data can be seen as a public good. You can own the data carrier but not the data.
The discussion about allocation is conducted between the following three points: 1. personality protection 2. fair and functioning data markets and 3. data subjects’ participation in the value of the data. The third point, however, is not valid as data is not produced and it is not hard work. The trade-off must therefore be found between the first two. And this requires social and political decisions. So why is “data property” a bad idea? You can sell a property. And it is a bad idea to sell data concerning your life, your habits and your personality.