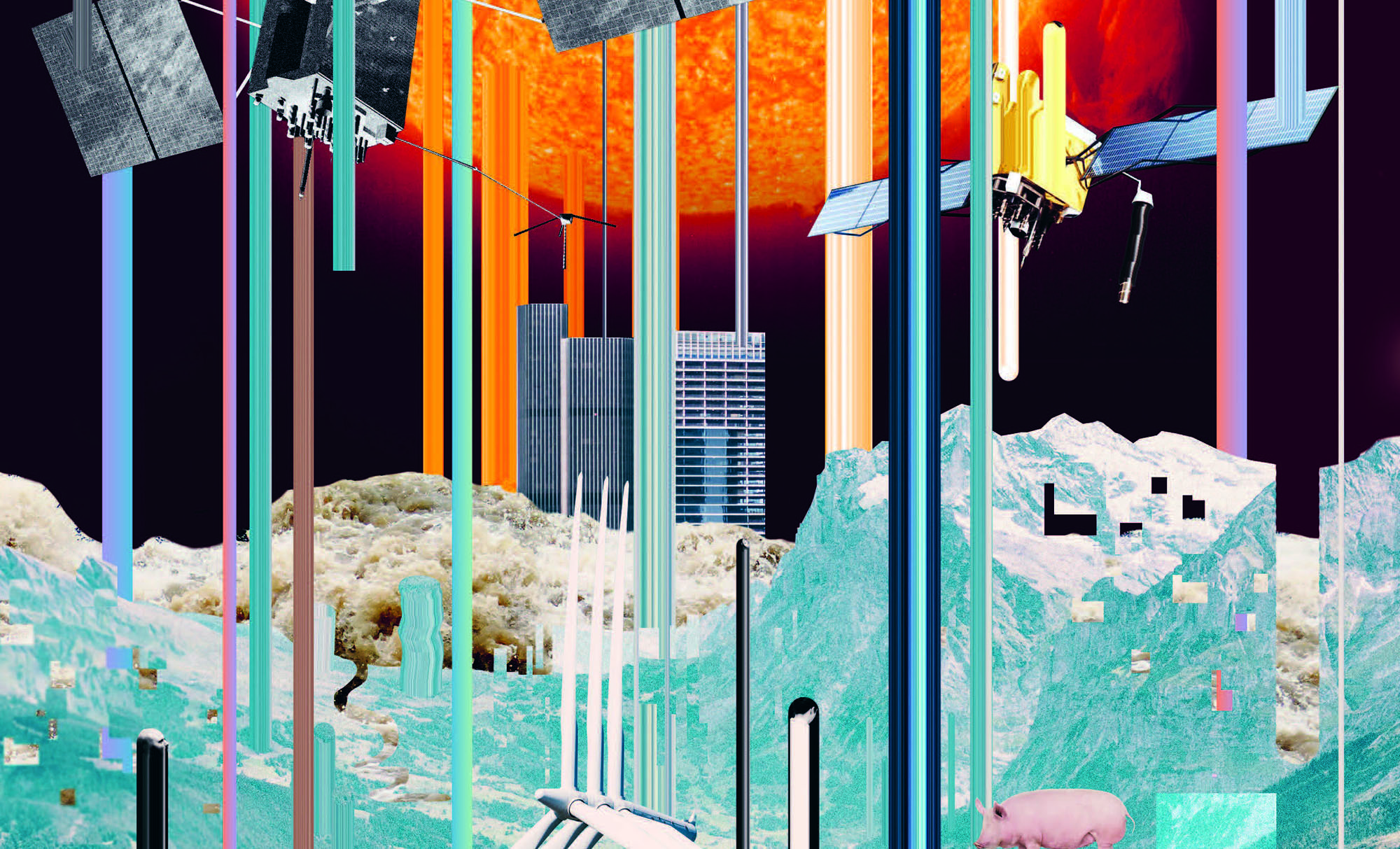
Big Data Applications
Big data applications bring opportunities in numerous domains. Their development requires, however, significant work: forming partnerships with stakeholders, securing technical and legal access to data, developing useful analytical models and validating the applications with users.
NRP 75 has developed applications based on big data in domains such as health, sustainability, socioeconomics, and scientific research. These represent only a small subset of the sectors that are exploring or integrating big data applications, which range from traditional data-intensive domains such as banking, marketing, and health, to new fields such as agriculture, journalism or policy making.
The projects funded by the programme improved existing methodologies and developed new ones for domain-specific big data applications and highlighted the potential benefits to society and the economy, such as the development of strategies for personalised medicine, smarter transport planning, integrated deployment of renewable energy and clearer evaluation of the effect of socioeconomic policies.
The various NRP75 applications have reached different stages of development, including initial models, prototypes and fully-fledged systems. This variety echoes the opportunities and challenges of creating practical applications for big data more generally.
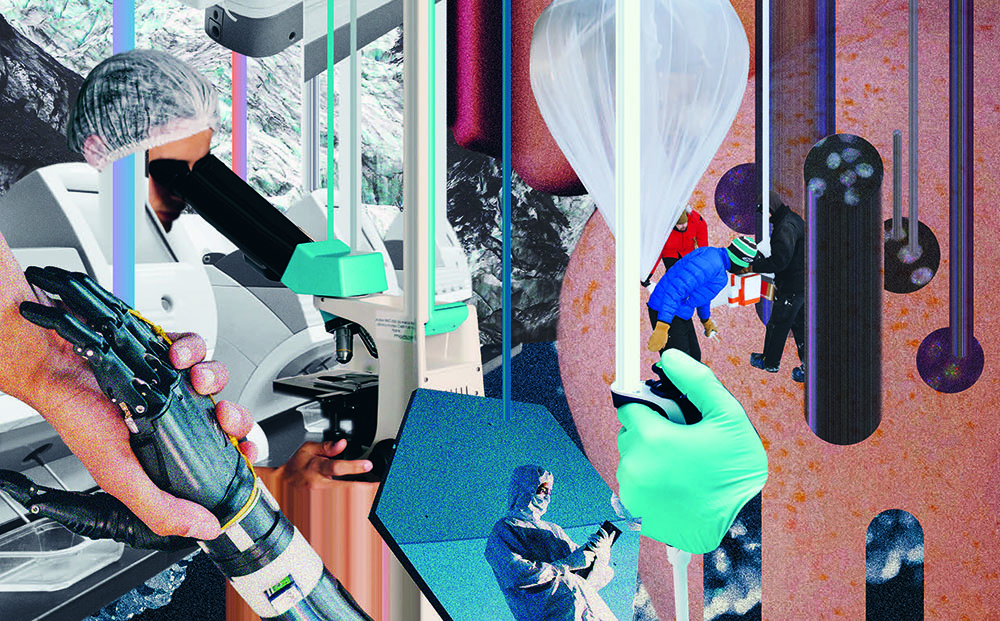
Improving and personalising healthcare
Numerous new approaches are pursued to tailor healthcare to the specific characteristics and needs of individuals and population groups. Applications of big data can potentially have a significant impact on health research, education and care, reaching beyond medical institutions into people’s homes.
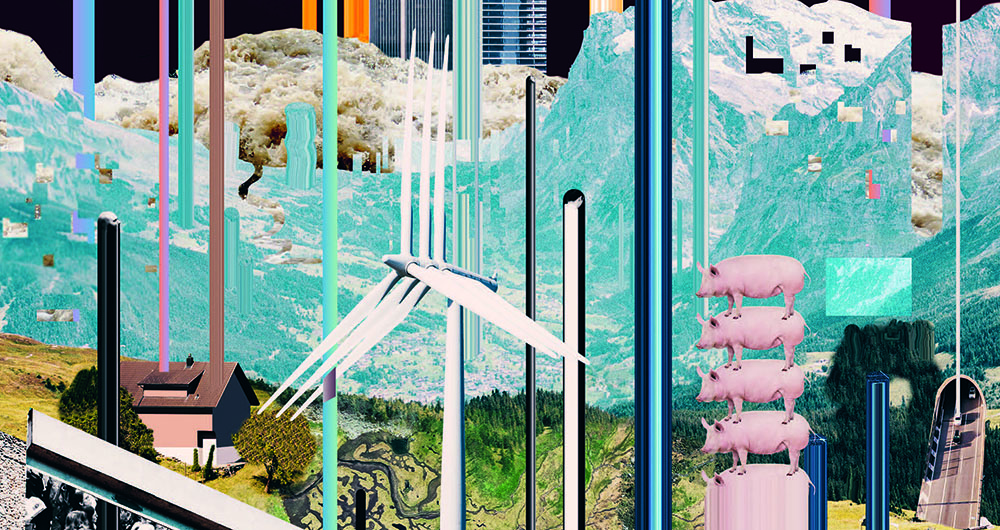
Supporting sustainability
Building sustainable societies requires optimising the interactions between the numerous components of energy, transport, supply or food systems.
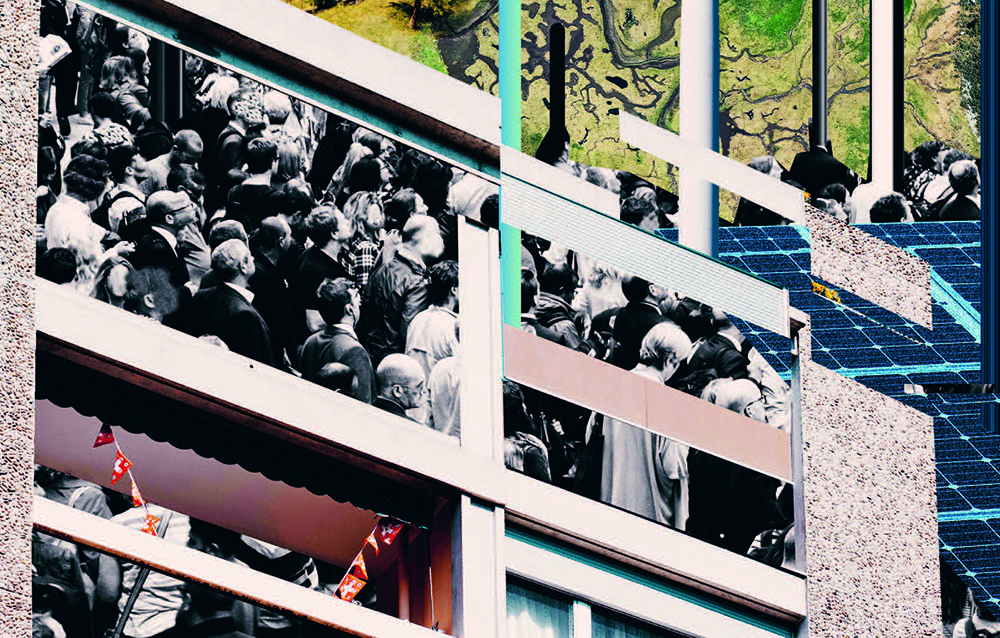
Better understanding of socioeconomic interactions
The fact that more data is being collected nevertheless offers many opportunities for new applications to help evaluate, inform and possibly improve policies.
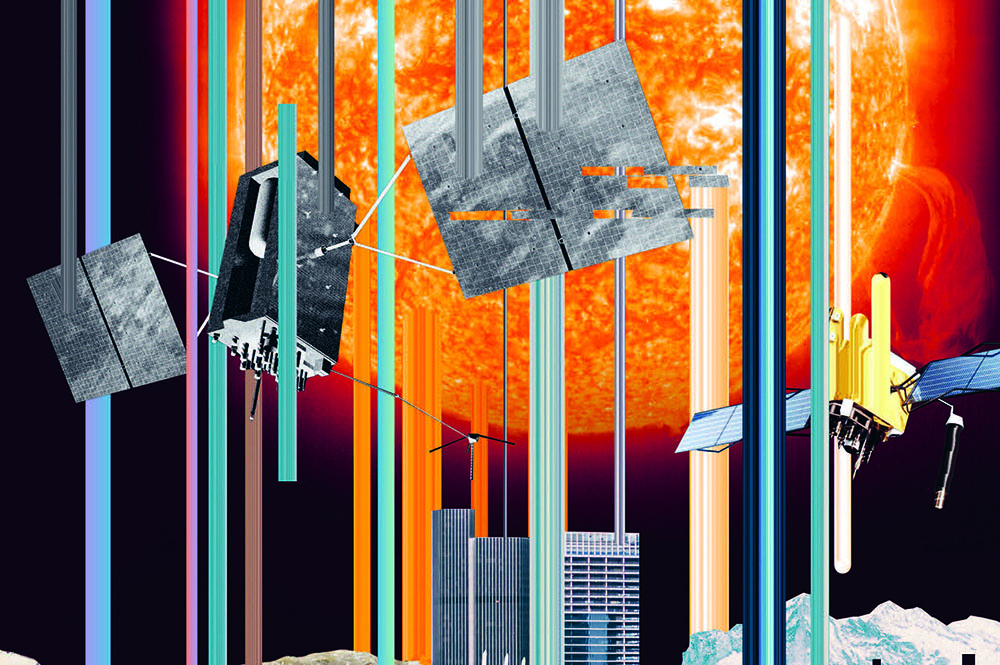
Accelerating research
Big data is also supporting innovation by playing an increasing role in fundamental research. This is seen particularly in very large international collaborations such as the experiments at the CERN in Geneva or, as well as in the high-throughput observational and analytical systems used for example in astronomy, satellite sensing or genomics.
Key messages on big data applications
Beware the hype
Reporting by the media, industry and think-tanks can give the impression that big data is a magic tool: just find the data, add some machine learning, train the algorithms, build an application, and you are ready to disrupt professional practices and entire industries. This overly simplistic vision hides numerous hurdles of a conceptual, technical, legal management and collaborative nature.
Building a big data application requires immense effort in many steps: creating partnerships with stakeholders; finding the data; assessing data quality, preparedness and completeness; storing the data securely, preparing the data for analysis; finding suitable existing algorithms and then adapting them or creating new ones; benchmarking the results; creating practical visualisations and interfaces to explore the results; and, finally, integrating the new application into established workflows.
Over-celebration of big data obfuscates very deep, if seemingly obvious, questions. Does the data exist at all? Is it accessible? Is it properly described with metadata? Can privacy be preserved and regulations respected? Do end-users actually need the intended application? Such questions must be considered at the outset in order to realistically gauge the amount of work ahead.
Is your domain big data ready?
Big data applications can be developed in a fairly linear way when they build upon existing prototypes (Renewable energy potential, Optimising transport management) or when high-quality and standardised data exist, as in weather, cartography and biological domains (Solar eruptions, Big genetic data). This allows computer scientists to focus mainly on technical issues, such as establishing a pipeline to access data in real time, building algorithms, or creating user-friendly interactive interfaces. NRP75 projects made a number of important advances in the design, implementation and evaluation of practical approaches to data engineering, including data management, analytics, visualisation, evaluation, auditing, integration and mining.
Conversely, it is much harder to build big data applications in domains that are less digitised, lack a data culture, and are averse or unused to sharing data (Pig data, Mapping global innovation). In such cases, significant time and effort is needed to deal with non-technical issues, such as setting up partnerships between stakeholders unused to sharing data. The availability and quality of data needs to be assessed early on, potentially leading to a revision of the application’s scope.
Big data demands interdisciplinarity
Managing datasets at the petabyte scale requires much time, manpower and collaborative effort to solve numerous technical and sociolegal challenges.
Building an application that makes an impact usually requires an interdisciplinary approach in which the various stakeholders address all potential issues and questions early on, including how the foreseen solution will be used by end-users (Pig data, Flood detection). Applied scientists, domain experts, industry partners, research engineers,- and ordinary citizens need to interact frequently to ensure that high-quality data are acquired and shared, as well as ensuring that applications meet the needs of their intended users. Scientists from NRP75 have explored new ways of interacting effectively with different stakeholders. Involving users early on improves the design of applications (Flood detection). This kind of experience ensures that academic research is able – when needed – to rapidly develop real-world applications.
The real world is messier than training data
Many machine learning algorithms can fail in the real world, with potentially grave consequences when it comes to health prediction or autonomous vehicles. This is a problem for supervised learning when algorithms are fed incomplete, noisy or unrealistically uniform training data. Turning to more frugal unsupervised learning could yield more robust systems (Flood detection).
Think privacy-by-design from the start
Issues related to privacy and regulation need to be addressed carefully, in particular with the help of legal experts. Privacy-by-design approaches should be considered and implemented as early as possible. This requires the careful consideration of overarching principles such as purpose limitation, transparency and proportionality, as well as data minimisation, accuracy and security (Intensive care units, City digital twins). Sharing experiences, within and across domains, helps establish best practice.