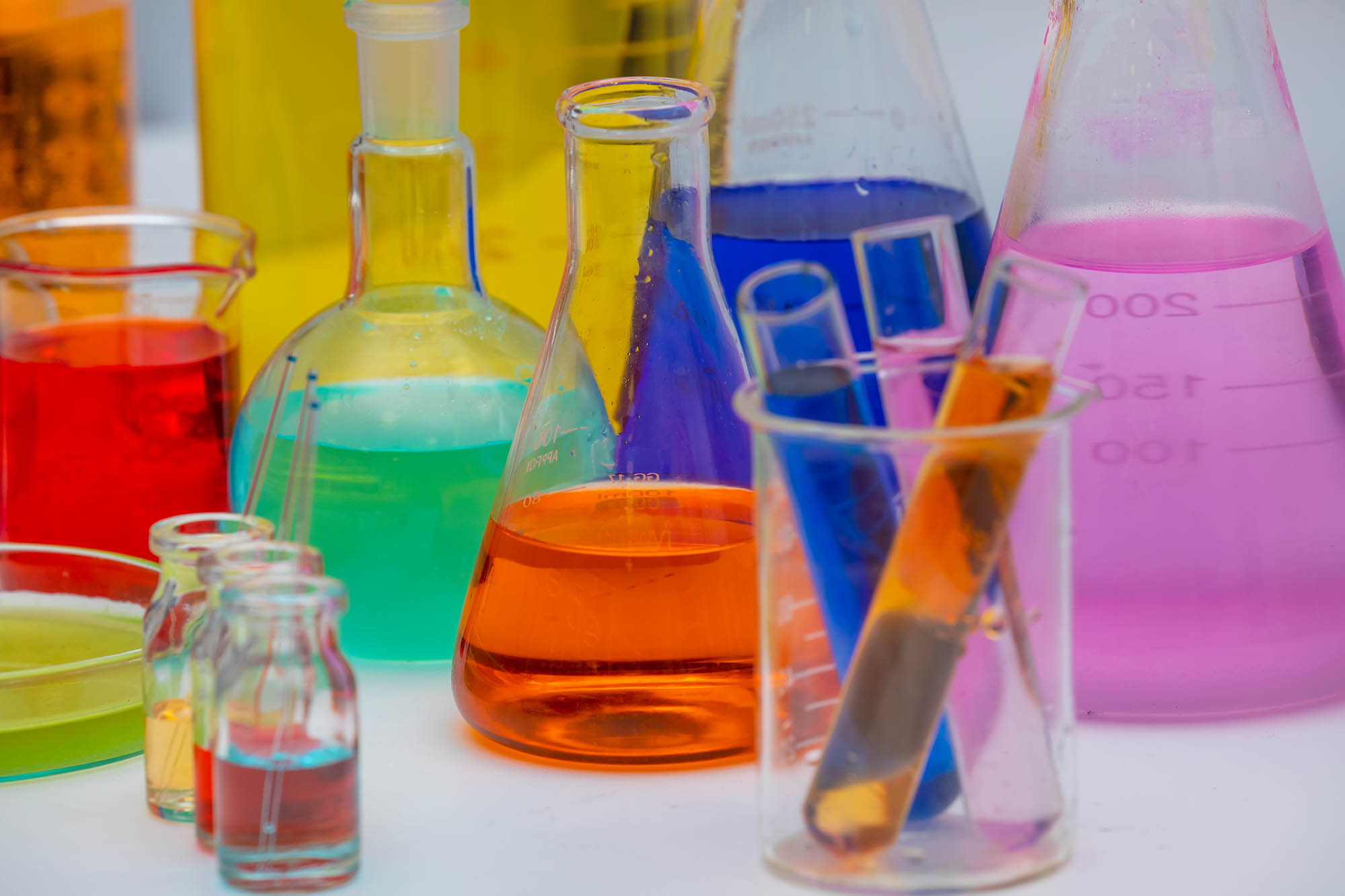
Computational chemistry: discovering new molecules
Author
Prof. Helmut Harbrecht
University of Basel
Interview with the principal investigators of this NRP75 project.
What was the aim of your project?
It takes a great deal of time and money to synthesise and test new materials in the chemical industry or new medicines in the pharmaceutical sector. This outlay could be reduced substantially if it were possible to control the complexity of chemical compounds. The aim of this project was to develop a highly efficient process capable of predicting the properties of chemical compounds. In this context, efficient means that the properties of any chemical compound can be predicted with great accuracy after an extremely short calculation time.
Results?
Three parts constitute the achievements within our project. The first part was the construction of training and test data. All data sets have been published and made available for scientific purposes. The second part was about quantum machine learning models which utilise multiple fidelities of quantum reference data of varying computational cost and accuracy. We were able to greatly improve the so-called learning curve, which means that our quantum machine learning model is much more powerful now. The third part were the mathematical foundation and the development of numerical methods for big data problems. These findings help to further improve machine learning models.
Does your project have any societal implications and recommendations?
Since chemical compounds do not have any personality rights, there is no societal implication by the present project. Nonetheless, there would be an economical implication, as the prediction of chemical compounds becomes possible and much cheaper.
Keyword “technology transfer”: In your opinion, who could be possible users of your project? Who could benefit from this?
This project provides experimental chemists with a new tool that can guide their efforts to identify, design, synthesise and characterise novel and interesting compounds by means of immediate predictions. In addition, the success of a model like this implies an improved quantitative understanding of the relationship between chemical structures and their properties. All our findings and all the results have been published and are hence available to everybody.
Big Data is a very vague term. Can you explain to us what Big Data means to you?
In the present project, “Big Data’’ corresponds to the chemical compound space. In principle, we can compute any desired compound, but it is impossible to compute all compounds. Therefore, we built a machine learning model to have a fast access to all compounds. Since, however, the chemical compound space is so large, we need an efficient strategy to choose the training sets in a clever way. It turned out that the computation of appropriate training sets is the bottleneck in the present project. This is a completely different setup compared to other “Big Data’’ problems where one already has a lot of data available which should be learned.